Accelerating drug development with machine learning
Researchers at the Cavendish have found that machine learning and artificial intelligence can significantly improve predictions of how therapeutic molecules will behave in the lab and in living things. Their results open a promising route to dramatically speed up the discovery and development of pharmaceuticals.
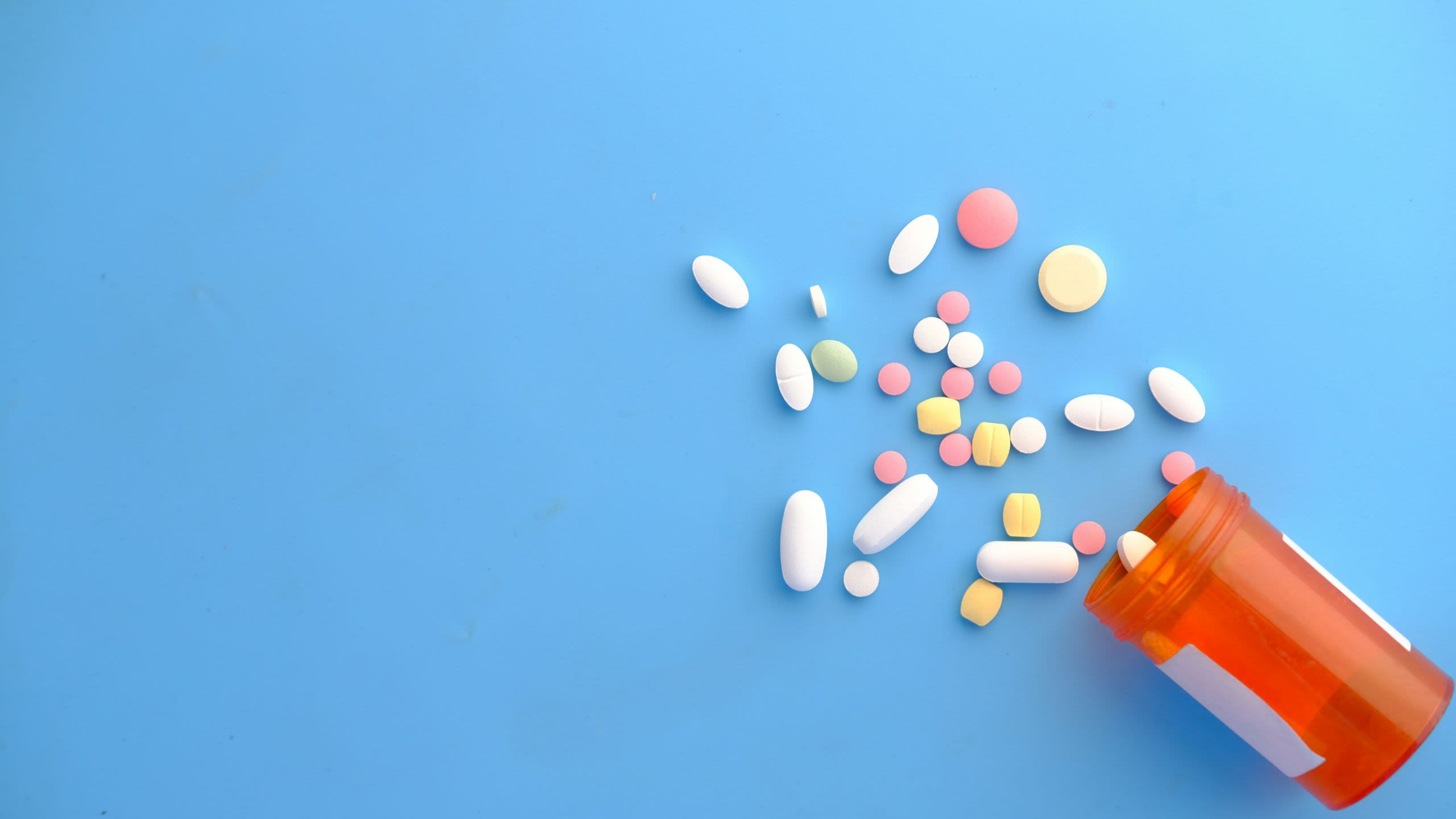
We have come a long way from chewing on willow bark to relieve fevers, but despite shiny new machines and billions of pounds poured into the development of new pharmaceuticals, safety testing, and chemical research, we still have a distinctly trial-and-error approach towards finding new drugs.
Even though we’ve made immense progress in understanding the human body’s biological pathways, we still struggle to predict how a foreign agent like a therapeutic will react in a complex biological system. In fact, we are often unsure how molecules will interact with each other even within a controlled
laboratory system like a test tube.
These limitations have big consequences for how we approach drug design: we don’t know ahead of time what the best molecule is to treat a given aliment, and even if we did, we don’t necessarily know how to make it in a reliable, let alone commercially viable way.
The historical discovery pipeline for therapeutics
Aspirin, one of the world’s most used drugs, was itself a modification of salicin, a natural component of white willow bark (Salix alba). Salicin was used as far back as 3,500 years ago as an antipyretic by the ancient Sumerians and Egyptians. To this day, drawing heavy inspiration from naturally produced molecules is still a common practice in drug discovery.
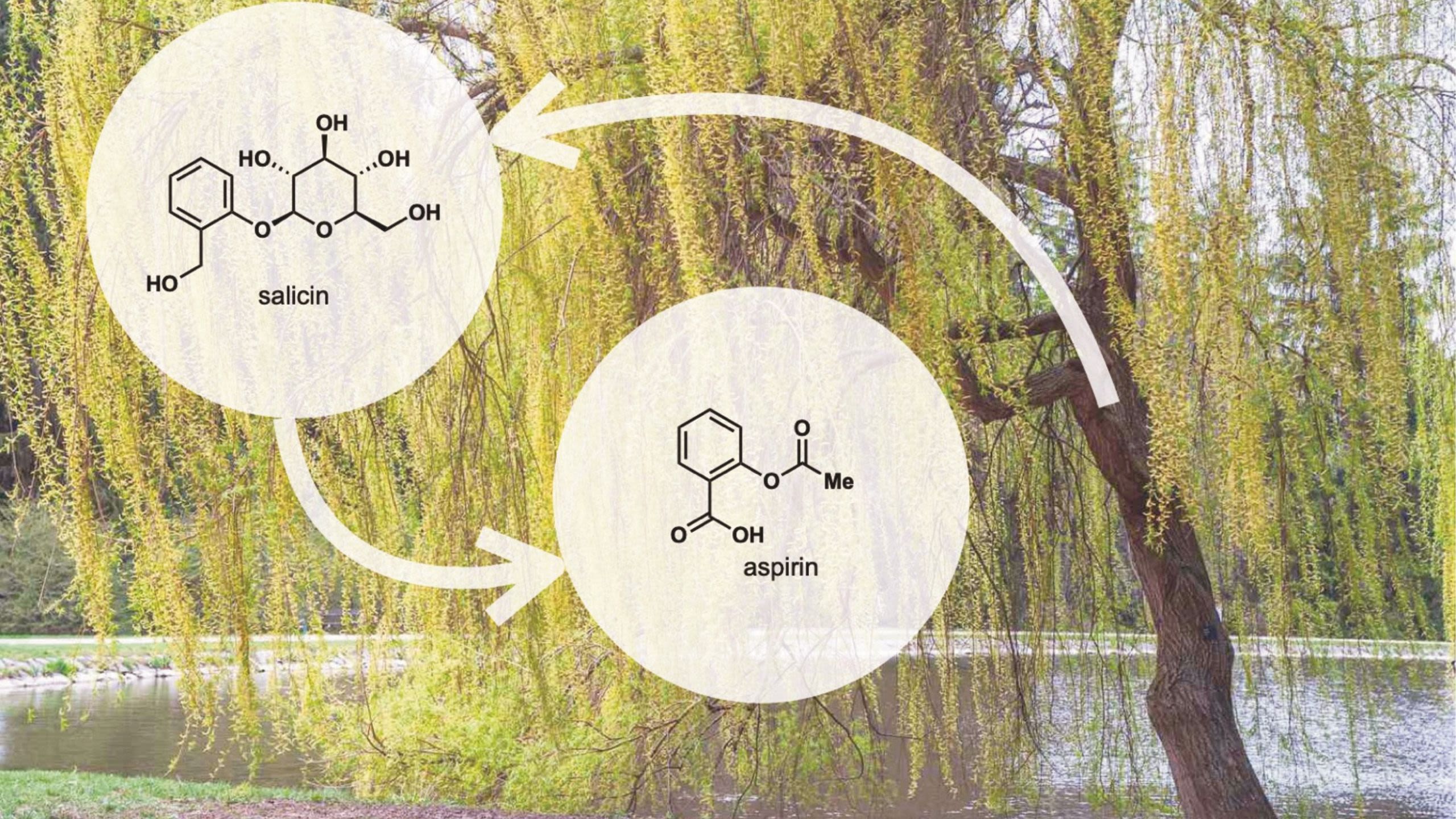
Understanding molecules in the test tube
A fundamental challenge is determining how molecules react with one another outside the human body. This is a crucial hurdle that must be crossed if we are to understand the intrinsic properties of the molecules we have already discovered and ultimately build new molecules with therapeutic applications. To that end, our team struck up a collaboration with Pfizer to investigate how molecules behave when exposed to specific types of reaction conditions. In particular, we focused on the ‘Minisci’ and ‘P450’ reactions – two chemistries with well-established utility in pharmaceutical research. Minisci reactions use radicals (molecules or atoms with an unpaired electron) to form new bonds with ring-shaped molecules, which appear commonly in nature and pharmaceuticals. P450 reactions also use radicals, and play a central role in drug metabolism and drug-drug interactions.
An overview of the Minisci and P450 reactions
An overview of the Minisci and P450 reactions
Given the recent breakthroughs in machine learning and artificial intelligence (AI) in biological research, such as AlphaFold’s ability to predict the structures of folded proteins, we suspected a machine-learning-powered framework could be the key to unlock new chemical insights. However, data-driven chemical research is plagued by a dearth of real-world experimental data. While biology has embraced a spirit of open-source, readily-accessible big data, data from chemistry is fragmented and more laborious to generate. Unfortunately, machine learning’s requirement for large data volumes is non-negotiable. Given the limited chemical dataset of around just 2000 reactions, we faced a daunting task.
A technique known as ‘transfer learning’ offered a possible solution. Here, a model previously trained for different task is repurposed, reducing the demand for a large training dataset. Our approach leveraged the fact that the original and new training tasks do not need to be very similar, just tangentially related for the model to perform well. Our model was first trained on abundant chemical information from one of the few open-source chemistry databases. Then it was re-trained on the much smaller Minisci and P450 reaction datasets to predict where on a molecule a new bond was likely to form during the reaction. To our delight, this tactic proved successful and highly accurate, outperforming the current gold standard (Fukui reactivity indices) as well as other machine learning based platforms.
Model performance of our machine learning approach versus Fukui reactivity indices. The grey dots indicate actual values for five repeated neural network initialisations, while ‘Best Model’ refers to the highest performing of these five.
Model performance of our machine learning approach versus Fukui reactivity indices. The grey dots indicate actual values for five repeated neural network initialisations, while ‘Best Model’ refers to the highest performing of these five.
Excitingly, it was then possible to apply this style of transfer learning much more broadly. By taking atomic position information from crystal structures supplied from the Cambridge Crystallography Data Centre, a foundational chemistry model was constructed. This model achieved state-of-the-art performance across a variety of important, unrelated chemistry tasks including predicting the yield of common chemical transformations, the lethal dosage of compounds (acute LD50), and even what a compound will smell like.
Understanding molecules in living things
However, the bigger challenge is understanding how these complex chemical systems will function in a living organism. Here, too, we have made some important strides. During the drug discovery process, each potential therapeutic drug is screened against a biological target of interest. Over the decades, the screenings have become more efficient, more automated, and faster. The overall workflow is to make the compounds, purify the compounds, give the compounds to the biological systems, and finally see what happens. Purification is often time consuming, and so researchers have devised a new process called ‘direct-to-biology’, which omits purification, going directly from compound synthesis to biological evaluation. Typically, this would lead to a muddy and confusing biological readout, but for select reactions that have been carefully optimised, it is a viable strategy.
Promising molecules for treating SARS-CoV-2 infection uncovered using our machine learning platform. SARS-CoV-2 main protease inhibition IC50 values shown below each compound.
Promising molecules for treating SARS-CoV-2 infection uncovered using our machine learning platform. SARS-CoV-2 main protease inhibition IC50 values shown below each compound.
Given the relative modernity of direct-to-biology, its scope of applicability is still narrow, but we suspected that a machine-learning-based approach could expand the reach of this powerful technique, effectively using our platform as a ‘de-confounder’ to allow chemical reactions deemed too noisy for direct-to-biology application to be included in the workflow. As a proof-of-concept, our team collaborated with members from the London Group at the Weizmann Institute of Science. We used our machine learning platform in combination with direct-to-biology screens to identify several promising molecules for the treatment of acute SARS-CoV-2 infection that would have been missed otherwise.
We still face many open challenges in the field of drug manufacture. Our research is part of a body of work that shows the value of machine learning as a tool for chemistry-centric research, allowing us to peel back the curtain on complex chemical and biological systems. As we look to the future of AI, exemplified today with the uncanny abilities of large language models such as ChatGPT, Claude and ERNIE Bot, one can only imagine where this marriage of machine intelligence and chemical modelling will take us.
Emma King-Smith is a synthetic chemist turned data scientist working at the intersection of machine learning and organic chemistry.
E. King-Smith et al., 'Predictive Minisci late stage functionalization with transfer learning', Nature Communications, 15, 426 (2024).
E. King-Smith, 'Transfer learning for a foundational chemistry model' Chemical Science, 15, 5143 (2024)
W. McCorkindale et al., 'Deconvoluting low yield from weak potency in directto-biology workflows with machine learning' RSC Medicinal Chemistry, 15, 1015 (2024).